
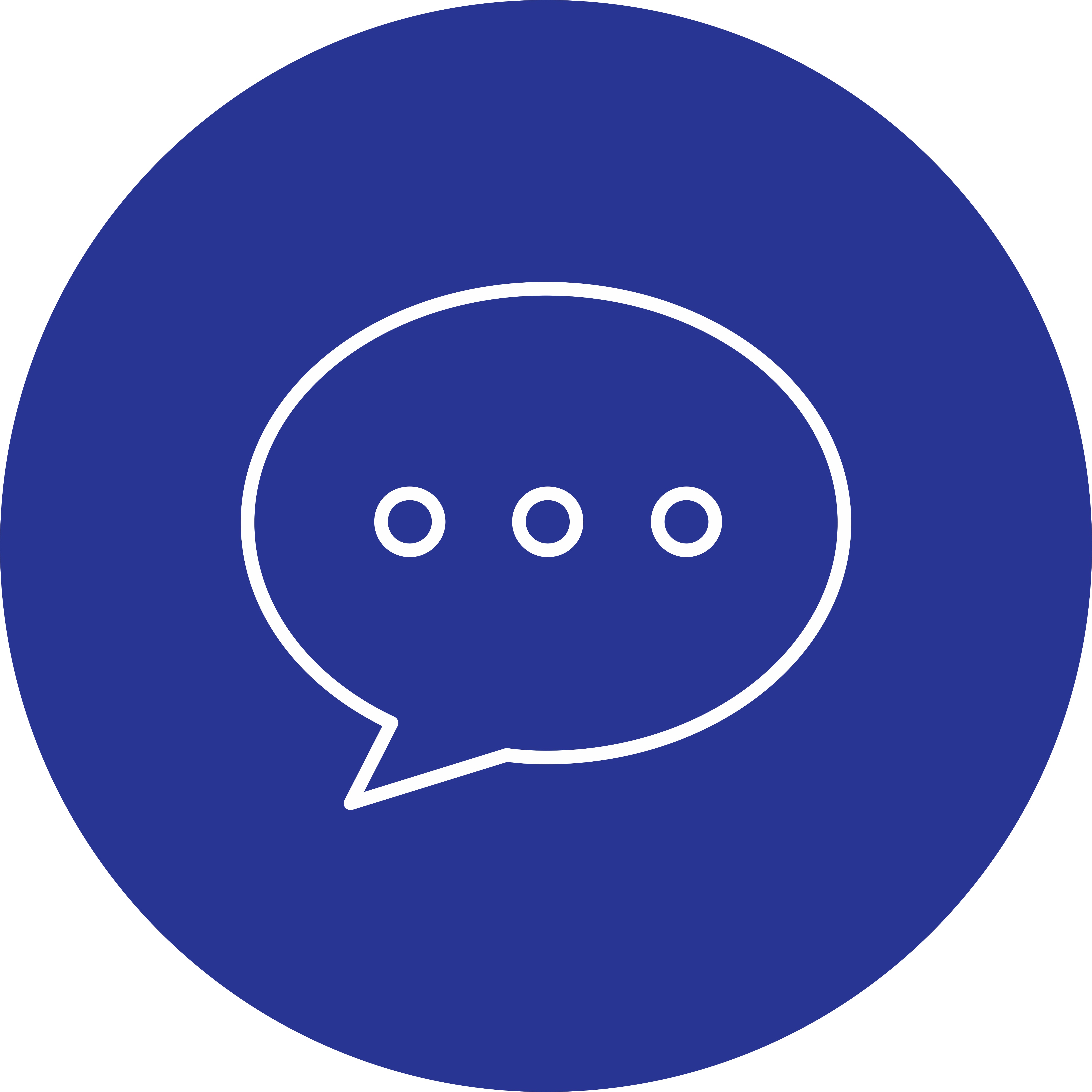
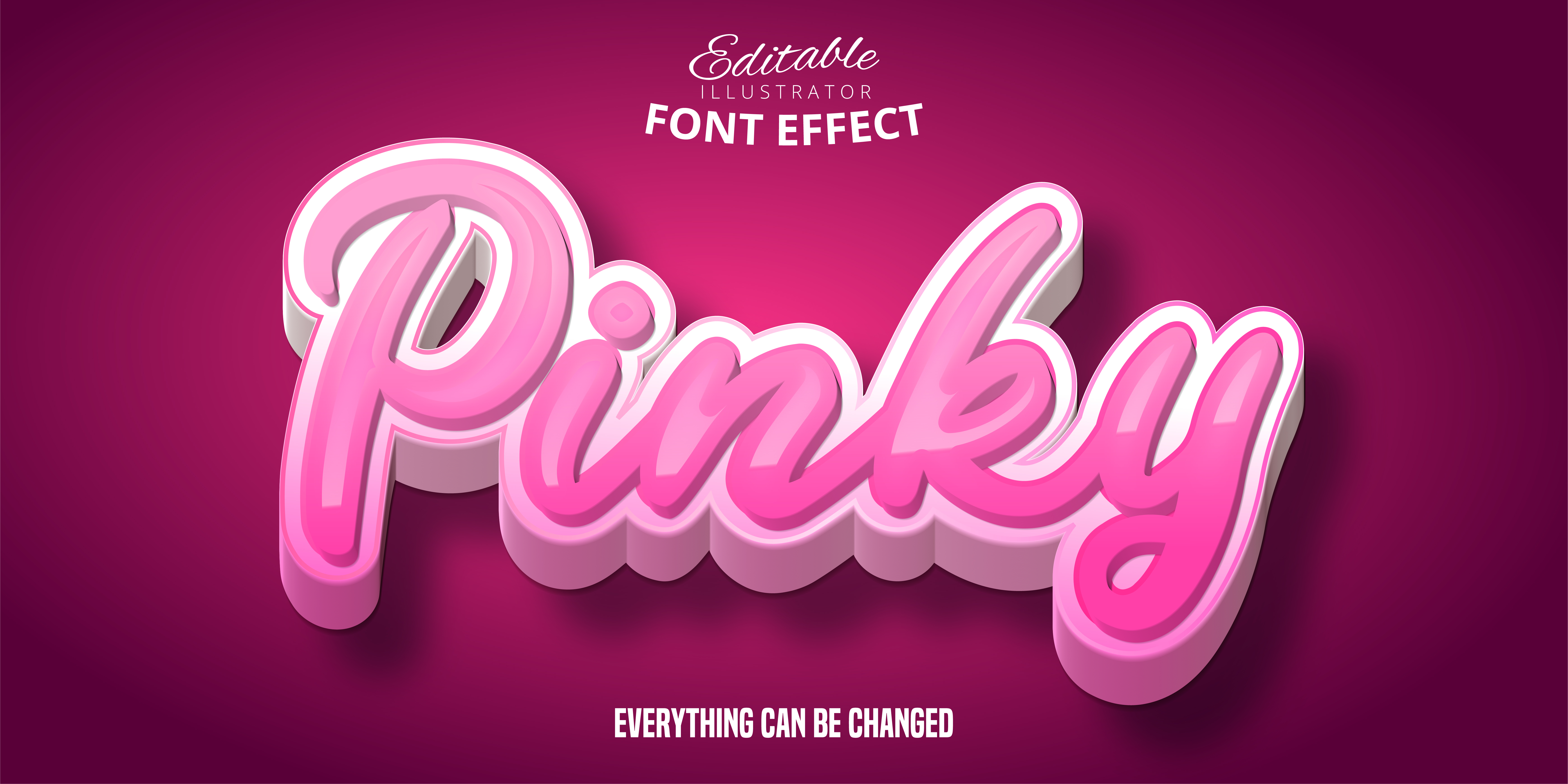
Therefore, it is difficult to reduce natural language to a structure that works well in most instances. There is typically a great deal of information in the arrangement of natural language, but it also typically varies a great deal in its arrangement from instance to instance. The challenge of the data structuring phase is to find some way to impose structure on data that allows meaningful information to be extracted.
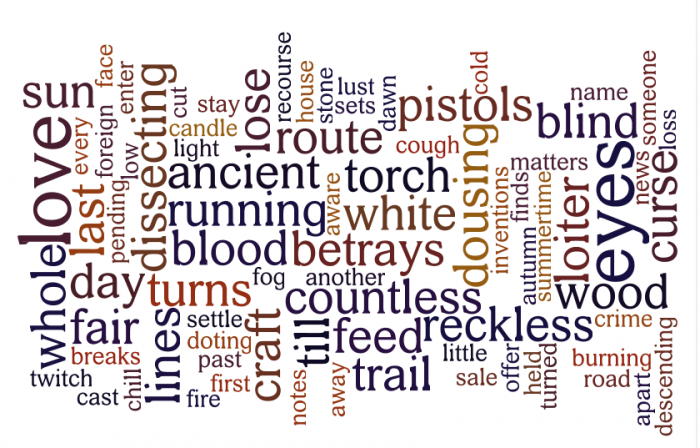
hormone receptor status for breast cancer patients). In the machine learning phase, the newly structured data are used to learn some characteristic of the data (e.g. In the data structuring phase, an algorithm attempts to impose some sort of regular structure on the data. Many NLP approaches are based on two main phases: data structuring and machine learning. Increasingly, NLP efforts have been focused on EHR to enable researchers to capitalize on its valuable information. NLP is not a specific method but rather a collection of approaches that involve extracting information from language as it is naturally spoken or written. This led many researchers to propose the application of natural language processing (NLP) techniques to these data 7, 8, 9, 10, 11, 12. Given the importance of clinical trials to drug development for a range of conditions, there is a critical need for methods that can automate and improve the efficiency of this process. Yet, particularly for clinical trials, this is currently the only available option at many institutions.
#VECTORIZE TEXT FREE#
Due to the volume of data generated by hospitals and other healthcare facilities, collecting data from free text is an arduous process to perform manually. Often, some of the most important information is stored in these fields, such as patient descriptions of symptoms, or clinicians’ observation of relevant signs of disease. Certain types of reports, such as pathology reports, are also entered as free text. Healthcare providers typically use freeform notes to capture important information when interacting with patients. The biggest challenge in the use of EHR comes from extensive reliance on unstructured data. However, the EHR was created for healthcare, not research, and the structure of the data leads to challenges in the research setting. EHR have also greatly simplified the process of recruiting subjects for prospective studies and clinical trials 6. For example, retrospective cohorts for exposures captured in patient records might relatively easily be assembled to study the impact of things like smoking or BMI on outcomes for diseases that are commonly treated at hospitals, such as cancer 5. In the era of big data, researchers quickly saw the potential to utilize EHR to advance their work in ways that were previously challenging 2, 3, 4, 5, 6. Many healthcare providers transitioned to EHR well in advance, and consequently, many hospitals now have years of patient records stored in a fashion that is relatively easy to search. Incentives were also provided to create and use electronic health records (EHR), which are more comprehensive, although the terms are frequently used interchangeably. Since 2015, the federal government has required most healthcare providers in the United States to use electronic medical records (EMR), or suffer penalties to Medicaid and Medicare reimbursement levels 1. These results suggest RWOV should be further developed for EHR-related NLP. AUC tended to be closer, but for HER2, RWOV was significantly better for most comparisons. For F1 score, RWOV had a clear edge on most tasks. RWOV performed as well as, or better than other methods in all but one case. As a proof-of-concept, we attempted to classify the hormone receptor status of breast cancer patients treated at the University of Kansas Medical Center, comparing RWOV to other methods using the F1 score and AUC. a relevant word occurs 5 relevant words before some term of interest). This facilitates machine learning algorithms to use the interaction of not just keywords but positional dependencies (e.g. RWOV is based on finding the positional relationship between the most relevant words to predicting the class of a text. Here, we propose Relevant Word Order Vectorization (RWOV) to aid with structuring. Natural language processing (NLP) can structure and learn from text, but NLP algorithms were not designed for the unique characteristics of EHR. However, much of the data contains unstructured text, presenting an obstacle to automated extraction. Electronic health records (EHR) represent a rich resource for conducting observational studies, supporting clinical trials, and more.
